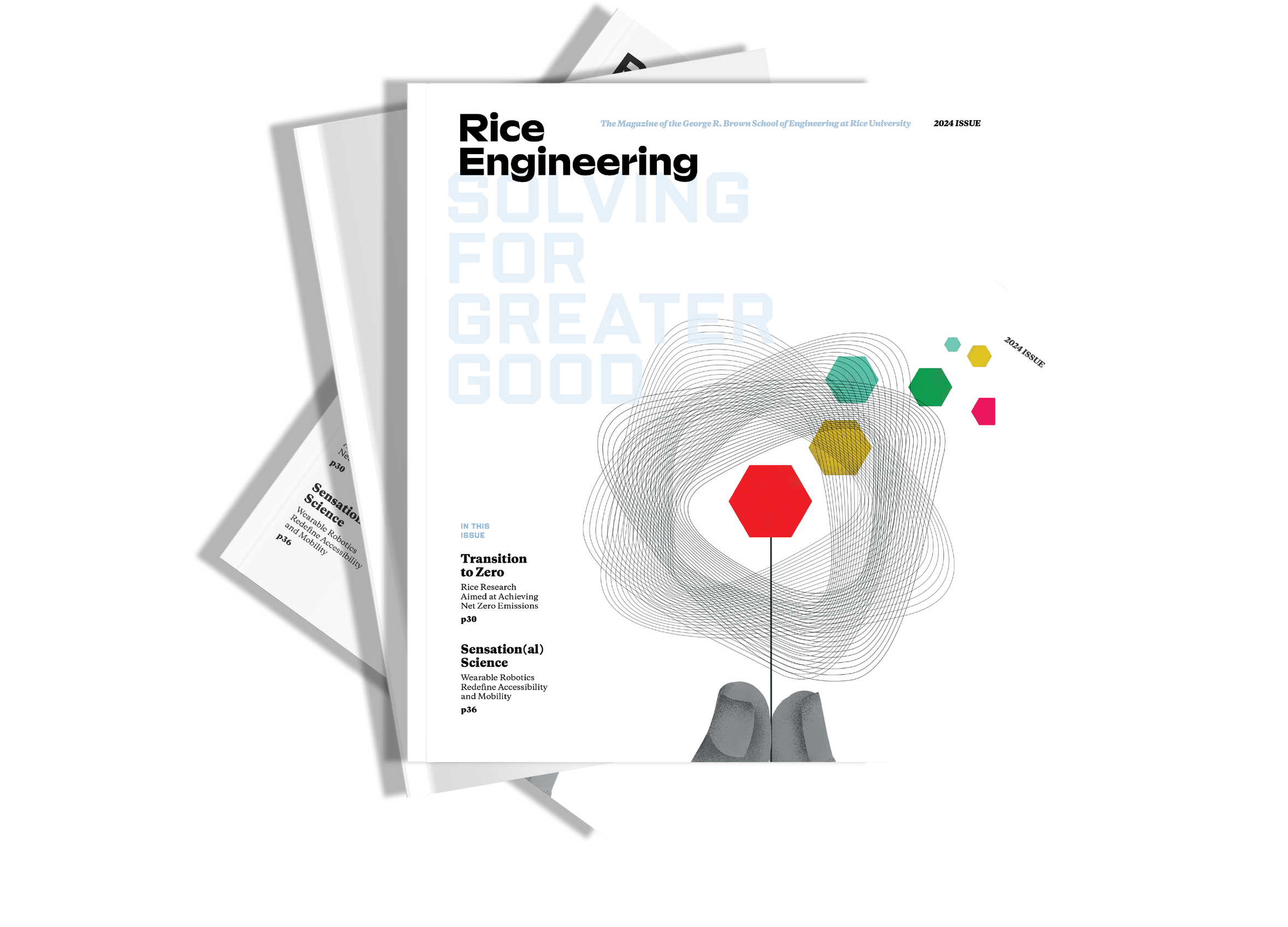
The spring 2024 issue of Rice Engineering Magazine is here!
At Rice Engineering, we are driven by a passion for innovation and a commitment to responsible engineering practices. It’s with great excitement that we unveil the new design of Rice Engineering magazine, which underscores our dedication to excellence in research, education, and service. The 2023-24 issue is full of news about how Rice Engineering is solving for greater good.
In Their Own Words
The Power of Prediction > Frederi Viens
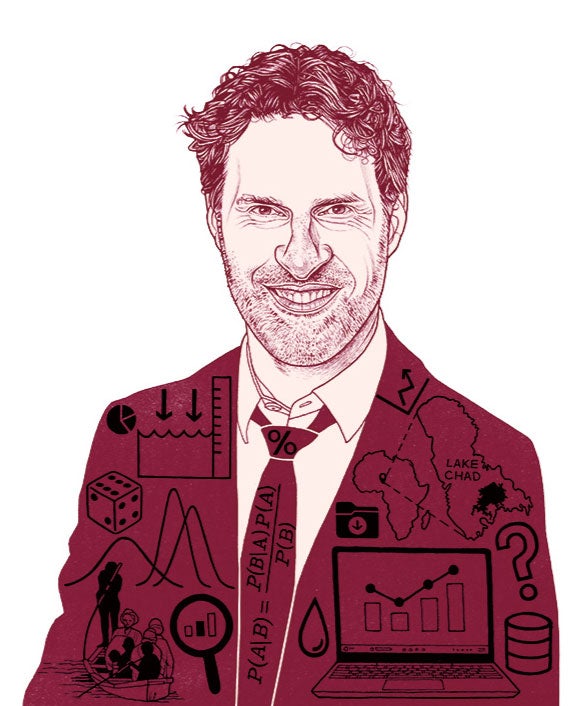
eng.rice.edu/lakechad
What is prediction? As a trained probabilist (yes, that’s a real word), I think of it as a way to compute the odds of some event happening, whether in a future experiment or in the future of our planet. What are the chances that the frequency of category-5 hurricanes making landfall in North America will increase to more than one per year by 2050? The public likes this kind of probabilistic thinking. It makes sense to them, the way it makes sense to professional statisticians. Mathematical modeling of what will happen in the future is so powerful that our brains think of it naturally, even without formal training in math.
The distinction between laypeople’s understanding of future odds and the way professionals understand it mathematically is the way in which we quantify uncertainty. Thomas Bayes, an 18th-century English clergyman and proto-statistician, understood that modeling uncertainty requires a leap of faith. Today we have the computational tools to implement his methods for formulating predictions.
To make a Bayesian prediction about future hurricane frequency, F, we need two things. We can relate F, for instance, to the atmospheric greenhouse-gas concentrations, C, in a linear model. We need to state how unsure we are about this model. We also need to specify our best guess for F itself, in the absence of any information about C, and how unsure we are. Based on past data for F and C, Bayesian statistics gives us updated uncertainty levels. Making predictions about F becomes straightforward, based on predictions about C. The cool thing about this example is that we have some control over the future of C. Because the model is so simple, it can be employed for uses other than prediction. Scientists could say that higher frequency of hurricanes is strongly associated with higher greenhouse-gas concentrations.
Statistical prediction based on quantified uncertainty is powerful but easily abused. Bayesian statistics has built-in guardrails against dishonest prediction, more so than standard “frequentist” statistics which places more restrictions on how the data behaves. Egregious errors can occur when misinterpreting correlations in the data. Public confidence in climate and environmental research erodes quickly when the word gets out that statistics might be used improperly. Much is at stake because strong statistical association — between climate change and hurricane frequency — is interpreted as attribution of one to the other. British statistician G.U. Yule explained in 1926 that the risk of abusive attribution is extremely high for certain data types which are common in the environmental sciences.
I am currently working on the mathematics behind this risk, known as Yule’s nonsense correlation, with Philip Ernst, a former Rice statistician now at Imperial College London.
We want to adjust correlation statistics so attribution can be gauged correctly. This problem is surprisingly challenging mathematically, illustrating how classical statistics should be handled with great care. They can be difficult to work with when the data doesn’t behave as expected.
Ernst and I obtained funding from the British Academy to study a hydrology question in the Lake Chad Basin region of northeast Nigeria using Bayesian statistics. Water-resource scientists published work two decades ago arguing that local farmers were causing damage to the ecosystem through small-scale irrigation. National governments and international aid agencies used this finding to recommend remediation that, in our view, would not be environmentally and socially sound. We think the original analysis suffered from Yule’s nonsense correlation. Our research will include public engagement events to discuss the dangers of misusing statistical tools and the ethical responsibilities of scientists, particularly in the context of Africa’s history after colonization. While this is a bit of a change for us since we’re usually focused on numbers, I believe that statisticians can make an impact by providing clarity in an uncertain world.