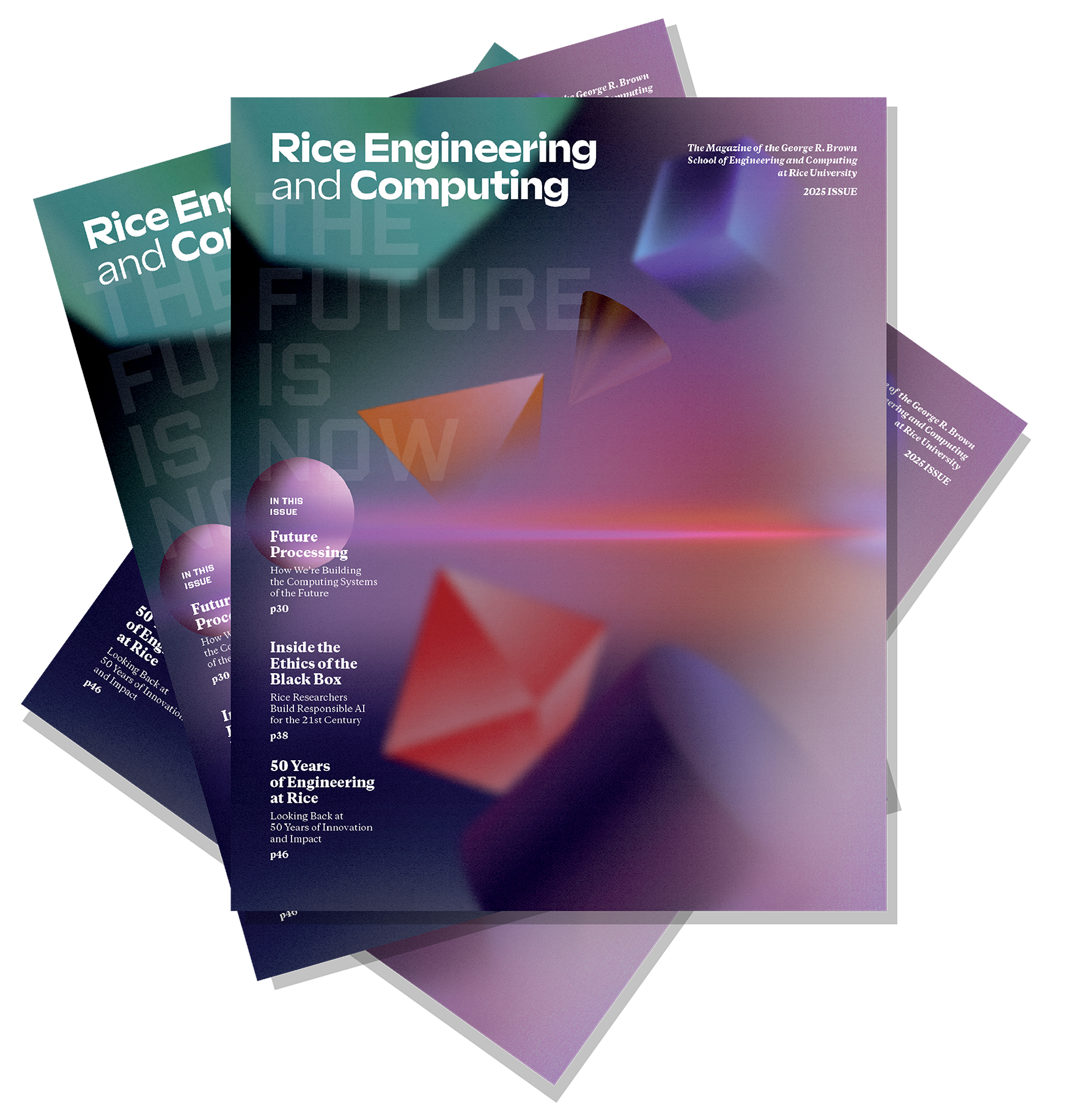
The 2025 issue of Rice Engineering and Computing Magazine is here!
In our 50th anniversary issue, we celebrate the deep and growing connection between engineering and computing. From our early breakthroughs in high-performance computing to today’s advances in AI and data science, Rice has long been at the forefront of computing innovation. This edition highlights some of the people, ideas, and investments shaping what’s next.
Building Responsible AI for the 21st Century
As the capabilities of artificial intelligence continue to grow, Rice Engineering and Computing faculty are providing leadership in responsible AI stewardship.
In recent years, the use of artificial intelligence (AI) in everyday life has exploded with tools such as ChatGPT and DALL-E. Beyond these, with recent advancements in technology, AI is revolutionizing a variety of industries – from energy and healthcare to communications and education. It plays a key role in tackling global challenges like climate change and advancing medical breakthroughs. In 2024, two Nobel Prizes were awarded for machine learning and AI-enabled breakthroughs. The Nobel Prize for Physics was awarded for the development of machine learning technology using artificial neural networks while the Nobel Prize for Chemistry was awarded for protein design and protein prediction. This recognition underscores the transformative power of AI.
With more than 20 faculty members dedicated to advancing the field, Rice Engineering and Computing is at the forefront of AI research. Now that AI is no longer a buzzword and the technology has advanced to the point where it is transforming the world in many ways, it is critical that we look at its impact on society, potential risks, and major ethical challenges while also embracing its vast potential.
“Since the advent of computing, researchers have had a tangible sense that one day it would be possible to endow machines with artificial intelligence and have since grappled with how to ethically and responsibly harness its power to benefit humanity,” said Richard Baraniuk, the C. Sidney Burrus Professor of Electrical and Computer Engineering.
Laying the Groundwork
The field of AI research emerged in the 1950s with the vision of creating machines with human-like intelligence. In the 1970s and 1980s, the U.S. government provided significant funding to make this vision come true, which led to the first AI boom. The initial push for its development came from national defense organizations who wanted an automated rules-based system to collect intelligence and translate conversations. However, “during that era, the field of AI overpromised and underdelivered and was replete with some famous failures,” said Chris Jermaine, the Victor E. Cameron Professor of Computer Science and chair of the Department of Computer Science.
These failures dampened the industry’s enthusiasm for AI research considerably and pushed the field into an ‘AI winter.’ Over the decades, as interest and funding for AI waxed and waned, other significant advances in computer science progressed in varied and seemingly unrelated ways. For instance, high-performance computing and the creation of artificial neural networks would later be adapted to future iterations of AI, helping to fuel its meteoric rise.
By the 2000s, there was renewed interest in AI research. Of particular interest was artificial neural networks – a type of machine learning paradigm that uses connected nodes and pattern-matching to process data in an attempt to mimic how a human brain learns. “What people realized is that you can train these networks, just like you can train a person to drive a car, or read a book, or do calculus,” Baraniuk said.
Other advances that contributed to a second boom in AI research were more powerful computers – specifically graphics processing units (GPUs), which were capable of much higher levels of computation – along with the introduction of enormous datasets that AI models needed to learn complex patterns and improve their accuracy. With an intricate system of artificial neural networks, increased computing power offered by GPUs, and ready availability of massive datasets, researchers could finally train the computers in a way that they acquired ‘artificial intelligence’ and were capable of mimicking human decision-making and performance.
Reducing AI’s Growing Energy Footprint
As interest in AI, along with its capabilities, continues to grow, several technical and ethical challenges lie ahead. A particular challenge associated with AI is its current energy requirements. Currently, AI is very energy-intensive, with ChatGPT’s daily energy consumption equivalent to that of hundreds of thousands of American households. “Energy production is only growing at the rate of 1 to 2 percent per year, but if AI continues to grow exponentially, at some point, the energy demands of AI will exceed our capacity for energy production, and that is a problem,” said Ramamoorthy Ramesh, professor of materials science and nanoengineering at Rice.
Moving forward, the challenge will be to reduce the energy demands of AI, which can be accomplished in several ways. The first strategy will be to come up with algorithms that require less computational power. “Can those algorithms be made a lot more efficient?” Ramesh posed. “We need to be discovering pathways by which we can make these computations a million times more efficient, energy-wise.”
Professor Anshumali Shrivastava specializes in using randomized algorithms to make AI more efficient. His work focuses on reducing the energy wasted by neural networks performing unnecessary computations, such as multiplying numbers close to zero that do not impact the result. By employing smarter algorithms, his research aims to enable AI systems to run on fewer resources, potentially replacing arrays of GPUs with a single CPU, which could significantly reduce the environmental impact of AI data centers.
This vision is at the core of ThirdAI, a company Shrivastava founded that develops software to make deep learning models run more efficiently on standard hardware, rather than relying on expensive, power-hungry machines. He also co-founded xMAD.ai, which uses his research advancements to speed up AI Agentic processes in industries that require high-performance computing while reducing energy consumption and, at the same time, are unhappy with AI Agents' reliability.
The second strategy will be to design hardware that is more energy-efficient. For this challenge, AI has the potential to solve its own problem, by facilitating the development of new materials that can power the hardware. “It’s a two-way street,” Jermaine said. “Materials scientists are going to build the materials that facilitate the next generation of AI, while AI acts as a foundational technology that accelerates the discovery of the next generation of materials.”
As we enter this new era of AI, its capacity to drive transformative progress across diverse domains opens new avenues for addressing today’s major challenges. “It’s an incredibly exciting time to work in this field,” said Lydia Kavraki, the Kenneth and Audrey Kennedy Professor of Computing and director of the Ken Kennedy Institute. “Developments are happening at a remarkable pace, and it’s truly inspiring to see just how quickly breakthroughs can emerge. Innovations we once believed out of reach are suddenly within our grasp.”
Bringing Transparency to AI Decisions
Another challenge associated with AI is that although scientists know AI can be developed by training the algorithms to remember and leverage previously occurring patterns in massive datasets to assemble new content or predict future events, they do not have a good understanding of the underlying mechanisms by which AI systems arrive at a specific answer. “While AI systems are somewhat accurate, they are prone to making mistakes and as of now, how AI produces its output with the input we provide is a black box, a mystery to scientists. This means if and when an AI system produces an inaccurate answer, scientists are unable to figure out what error led to the mistake. It’s simply not possible to audit or correct the decisions it makes,” Baraniuk said.
“It’s like when you have a human driver that gets into a car crash but is uncooperative or unable to describe what happened and why he took the actions he did. We need to put systems in place to prevent such crashes from happening in the future,” Baraniuk said. “To do this, we need to understand what’s going on inside the black box.”
If we are to rely on AI to make crucial life-altering decisions, such as spotting a tumor in an X-ray or generating accurate information for consumers, then we ought to be able to identify and correct errors when they occur. “The major challenge will be developing systems that are responsible and that humanity can build trust with,” Baraniuk said. To address this challenge, Baraniuk and his collaborators are working on understanding what is happening within that black box, so that they can better understand the strengths and limitations of AI.
One tool Baraniuk’s team has developed is called SplineCAM, which acts like a CT scan for deep neural networks, enabling researchers to measure their inner workings. Already proving its value, SplineCAM identifies and quantifies changes taking place within a deep network when an emergent behavior occurs.
Correcting AI’s Blind Spots
A critically important challenge in AI is the creation of systems and algorithms that are unbiased. “There are deep technical questions about the mathematical definition of fairness and bias in AI,” Jermaine said. “This represents a very deep set of computational questions that we have to be aware of. How do you develop AI tools that don’t lie, cheat, steal or exhibit prejudice?”
Modern AI is created by training artificial neural networks on very large datasets. However, if these datasets are biased, then the resulting AI will also be biased. This presents a number of challenges in multiple areas of research, whether it is addressing the existing biases in healthcare, education or the energy sector. For example, if AI is trained on current health research, which has been shown to have biases relating to conditions associated with either women or minorities, then the result will be misdiagnoses or the perpetuation of already existing inequities.
“AI is exposed to the totality of human-generated data, so it can create content or make predictions on prior patterns. It essentially mirrors humanity’s best attributes and perpetuates our worst flaws,” Jermaine said. “Humans not only use ‘cause and effect’ logic in our decision-making but also philosophy, values, and ethics to make holistic decisions that hopefully bring out the best in us and temper our worst instincts, which AI cannot do yet.”
Moving forward, addressing biases in AI will require addressing the biases found in datasets, such as correcting for the underrepresentation of specific types of data, while also creating systems that can temper these deficits.
Associate professor of computer science Vicente Ordóñez-Román aims to reduce bias in natural language processing and computer vision. His research group creates algorithms that detect and correct the amplification of biases present in training data, especially in image datasets that frequently depict stereotypical situations. Ordonez hopes to develop more equitable systems for uses such as facial recognition, content recommendation, and medical diagnosis by exposing AI models to a wider range of data.
Ben Hu, an associate professor of computer science, is focused on enabling transparency and improving trustworthiness of AI without sacrificing performance. His research explores how reinforcement learning techniques can be used to dynamically adjust AI decision-making processes, allowing models to self-correct when biased patterns are detected. Hu's work provides solutions for creating more ethical, safe, and adaptive machine learning models, especially in health care, finance, and public policy.
Baraniuk’s research group has also developed MAGNET and Polarity Sampling, two tools designed to tackle bias in generative AI. MAGNET fine-tunes models to create a more balanced representation of the dataset, while Polarity Sampling adjusts outputs from pre-trained deep generative networks to increase fairness, ensuring AI-generated content better reflects diverse perspectives.
Solving Global Challenges Using AI
The Ken Kennedy Institute was founded in 1986 with a dual mission: to advance computing research and to spark interdisciplinary collaborations that leverage computing as a transformative force. Today, the Institute champions foundational AI research and partners with experts across science, engineering, and medicine to co-create solutions finely tailored to the pressing challenges of our time.
One of the foremost objectives of the Ken Kennedy Institute is to push the boundaries of AI while addressing its core limitations - bias, interpretability, privacy and security, resource and energy consumption, generalizability and robustness, and, critically, alignment with human values. “In our work, we strive to create methodologies that don’t simply patch these vulnerabilities but account for them from the earliest design stages,” said Lydia Kavraki, the Kenneth and Audrey Kennedy Professor of Computing and director of the Ken Kennedy Institute. “That way, responsibility principles are baked into the technology from the outset rather than tacked on as an afterthought.”
Toward this goal, the Institute supports teams focused on algorithmic and system frameworks for generative AI with reduced energy consumption; next-generation AI methodologies in optimization, graph problems, online learning, and deep neural networks for scaling computations; frameworks that integrate physical modeling with data-driven AI for robustness and efficiency; novel computer vision and robotics approaches for dynamic and interactive environments that require real-time adaptation; and AI-human collaboration.
“We also thrive when we collaborate with domain experts on real-world applications,” said Kavraki. “It’s immensely rewarding to see AI accelerate breakthroughs in other disciplines, and at the same time, these applications reveal what we still can’t solve, pointing us toward the next major advances in AI.”
To foster interdisciplinary innovation, the Institute backs teams developing AI methods for understanding climate risks and enhancing infrastructure resilience. It also supports AI-driven computational biology research for cancer screening and early detection, early-warning systems for pathogen outbreak tracking, and improved vaccine and drug design.
Using AI to Customize Education
In 1999, Rice Engineering and Computing faculty member Dr. Richard Baraniuk founded Connexions with the goal of making education accessible for all. Since then, Connexions has expanded into multiple platforms, including OpenStax, which publishes free, high-quality textbooks; OpenStax Assignable, which helps students with practice and assessment; OpenStax Research, which is focused on innovations in education, and OpenStax CNX, which is one of the first and largest open education platforms.
Now, with improved AI capabilities, Baraniuk and collaborators are working to make education more accessible, offering a personalized approach to learning that can take a student’s interests and talents into account when crafting educational materials.
“We are creating AI education tools that can offer students a curated, multimedia experience that is tailored for them,” Baraniuk said. “We’re moving from a textbook age into a personalized multimedia age.”