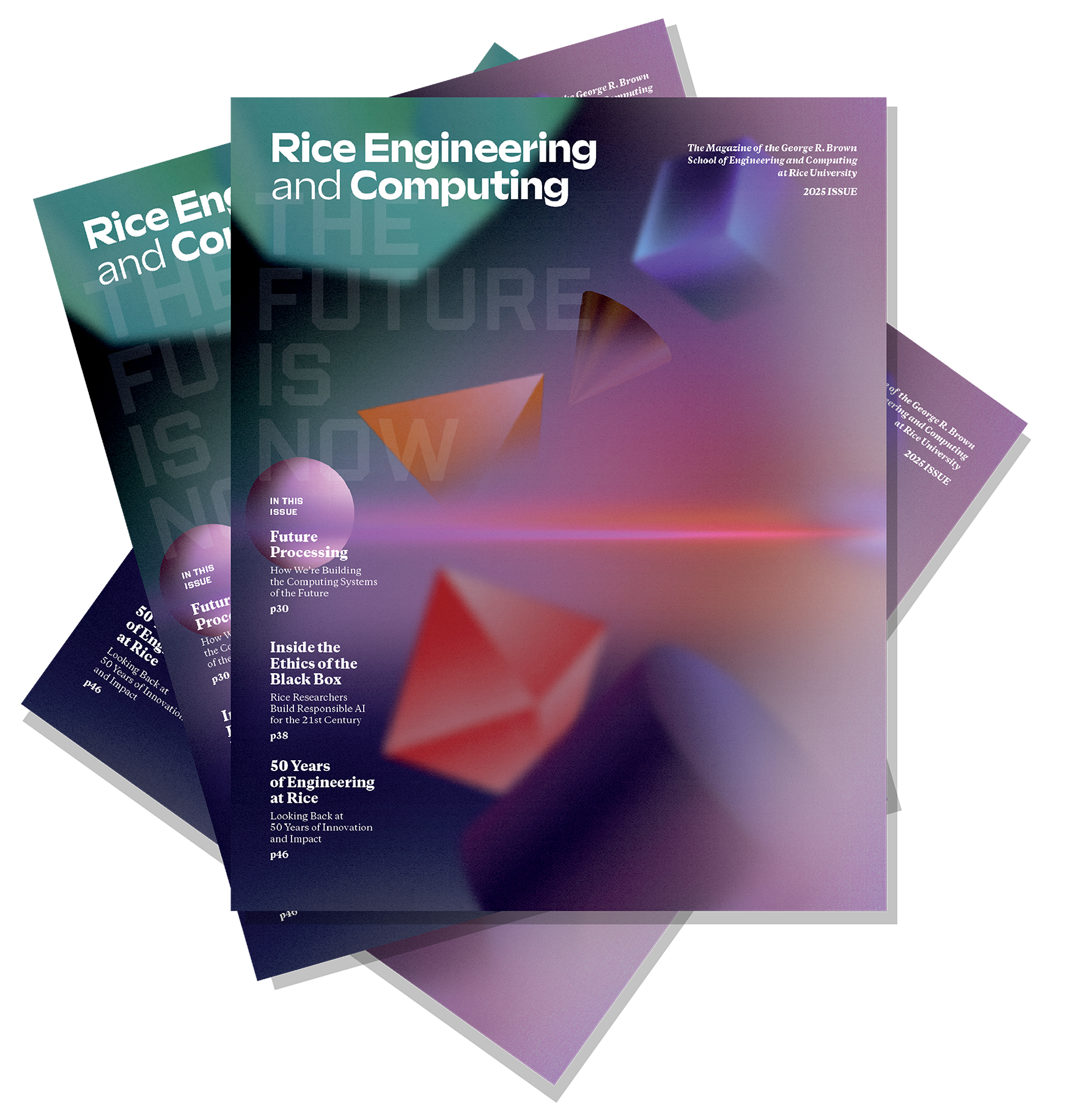
The 2025 issue of Rice Engineering and Computing Magazine is here!
In our 50th anniversary issue, we celebrate the deep and growing connection between engineering and computing. From our early breakthroughs in high-performance computing to today’s advances in AI and data science, Rice has long been at the forefront of computing innovation. This edition highlights some of the people, ideas, and investments shaping what’s next.
Computing + X: Rapid Advances in Computing Transform Science, Health, and Energy
The last 50 years have seen massive growth in computing technologies and its near ubiquitous presence in our daily lives. The rapid advancement in generative artificial intelligence (AI) models like ChatGPT has ushered in a new era where AI and robotics are expected to transform our lives further. Take a look at how Rice researchers are harnessing these technologies to accelerate scientific discovery, revolutionize healthcare, and create sustainable solutions.
Solving for Science
Scientific research generates extensive amounts of data. Computational and AI-driven approaches facilitate and accelerate data mining, extraction, and analysis, enabling faster, more accurate discoveries, simulations, and hypothetical frameworks for further experimentation.
Rice computational biologists, Luay Nakhleh, William and Stephanie Sick Dean of the George R. Brown School of Engineering and Computing and professor of computer science and biosciences; Vicky Yao, assistant professor of computer science; and others are advancing biomedical research and clinical care. Their work focuses on developing tools that help scientists and physicians trace evolutionary origins, pinpoint precise mutations, and dysfunctional cellular processes underlying complex diseases like cancer and Alzheimer’s.
Seismic, a new computational framework developed by Yao and her team, is an example. It integrates data from granular single-cell studies with population-scale Genome-Wide Association Studies (GWAS) data. “By combining data modalities that are typically analyzed separately, Seismic gives biologists a higher resolution perspective of a disease that facilitates deeper exploration and paves the way for targeted diagnostics and therapies,” Yao said.
Moreover, leveraging computational and AI tools to diagnose, monitor, and treat complex diseases like cancer has led to treatments that improve patient outcomes and quality of life. “Such multidisciplinary approaches have led to many disease-specific personalized biomarkers, diagnostics and treatments. When combined with evolutionary insights, they often result in powerful ‘out of the box’ solutions,” Nakhleh said.
A team led by Todd Treangen, associate professor of computer science and Ken Kennedy Institute’s AI2Health cluster lead, has created AI-powered open-source software tools such as SeqScreen to rapidly analyze vast amounts of genomic and metagenomic data from environmental and patient samples to detect novel bacterial or viral pathogens that might pose emerging risks to public health. “With AI-powered approaches for designing synthetic DNA and proteins, there is a critical need for improved screening tools to prevent the accidental creation of infectious proteins,” Treangen said. “So, we are also developing computational tools to screen for sequences of concern and thus, avoid unintended harm.”
Interestingly, despite widespread use of AI, it is still a mystery how machine learning algorithms make decisions. Associate professor of computer science, Xia (‘Ben’) Hu, and team are cracking open this ‘black box’ and applying their findings to construct more reliable AI models. “Choosing the right AI model for a specialized task is complex and daunting,” Hu said. “Our goal is to make this process transparent and accessible so domain experts (e.g., physicians) without expertise in machine learning algorithms can also custom design and deploy the right AI model for their specific need in the future.”
Solving for Health and Well-being
From routine conveniences like virtual telehealth appointments to cutting-edge innovations like AI-driven diagnostics, robot-assisted surgeries, and drug discovery platforms, automation has transformed healthcare in recent decades. The latest advancements in AI technologies are expected to further revolutionize medicine and public health.
Computational and AI-driven tools are critical for early detection and continuous monitoring of epidemics. During the COVID-19 pandemic, wastewater surveillance emerged as a powerful predictive method for accurate and unbiased forecasting of location-specific trends in community infections. Katherine Ensor, Noah G. Harding Professor of Statistics, Lauren Stadler, associate professor of civil and environmental engineering, and Loren Hopkins, professor in the practice of statistics and chief environmental scientist at the Houston Health Department, established a citywide wastewater monitoring system and implemented a statistical framework to assess levels of SARS-CoV-2 and variants of concern in wastewater that informed public health responses. Todd Treangen and Lauren Stadler expanded this ongoing effort to develop computational tools to design sensitive assays to detect other emerging biothreats through wastewater surveillance. “Through collaborations with Houston Wastewater Epidemiology, the Houston Health Department, and Houston Public Works, we can now detect dozens of infectious diseases including measles, flu, RSV, mpox, and others,” Stadler said.
Rice researchers are committed to using computational and AI-driven approaches to make healthcare affordable and accessible. Recently, a team led by Peter Lillehoj, Shankle Chair in Mechanical Engineering and associate professor of bioengineering, and Kevin McHugh, assistant professor of bioengineering and chemistry, developed a low-cost, AI-enabled microfluidic flow cytometer, a device that counts and identifies cells from unpurified blood samples. “This lab test can now be performed anywhere, including rural and resource-limited settings, for a fraction of the cost, and we envision it will be useful for many new point-of-care clinical and biomedical research applications,” Lillehoj added.
Biological drugs, such as monoclonal antibodies, recombinant proteins, or gene therapies, offer many advantages over traditional therapeutics. However, their complex absorption, distribution, metabolism, and excretion behaviors make it challenging to find the most effective delivery methods and dose regimens. Associate Chair of Bioengineering and professor of bioengineering and biosciences, Oleg Igoshin and his team have constructed computational models to analyze and improve our understanding of these processes in both preclinical and clinical studies, to predict doses for clinical trials, and to understand the complexities associated with different dosing strategies.
Solving for Energy
Widespread automation has led to an ever-growing need for plentiful, sustainable and reliable sources of precious chemicals and energy.
The recent surge in AI has allowed chemists to quickly screen several potential materials to identify the best catalysts for electrocatalysis, a sustainable method to manufacture chemicals. Since few atomic-scale tools are available to visualize ongoing chemical reactions, chemists rely heavily on computational models to simulate a catalyst’s internal three-dimensional lattice structure and the reaction environment. “We’ve collaborated with Thomas Senftle, the William Marsh Rice Trustee Associate Professor in Chemical and Biomolecular Engineering, to develop molecular models that simulate a catalyst’s surface properties, its activity, and key reaction mechanisms — which coupled with AI-driven data analysis — has allowed us to develop new and improved catalysts much faster than before. We’ve also partnered with Meng Li, Noah Harding associate professor of statistics, to build AI models to explore reaction conditions that can extend battery life,” said Haotian Wang, associate professor in chemical and biomolecular engineering, materials science and nanoengineering, and chemistry.
To develop efficient, low-cost methods for enhanced gas recovery and subsurface carbon dioxide and hydrogen gas storage, Rice chemical engineers, led by Walter Chapman, William W. Akers Professor, Chemical and Biomolecular Engineering and Philip Singer, assistant research professor in chemical and biomolecular engineering, have developed computational models to study fluid behavior in confined environments. Their research employs quantum and molecular simulations, as well as statistical mechanics-based theories (iSAFT), to model fluid behavior in energy applications. “A key advancement is a computational approach we’ve developed to predict nuclear magnetic resonance (NMR) relaxation without adjustable parameters, which provides molecular-scale insights into how fluid partitions and is transported through porous media like rocks,” Chapman said. These insights have also helped them develop new MRI contrast agents for biomedical imaging in collaboration with Amanda Marciel, assistant professor and William Marsh Rice Trustee Chair of chemical and biomolecular engineering.
Ming Tang, associate professor of materials science and nanoengineering, is developing computational approaches to improve the efficiency, lifespan, and safety of lithium-ion batteries, which are crucial for renewable energy storage in electric vehicles (EVs) and personal devices, and the integration of intermittent sources like solar and wind power into the grid. With seed funding from the Ken Kennedy Institute, he and Xia (Ben) Hu, are also exploring the use of AI to assess the degradation levels of EV batteries and their individual cells. “EV batteries with less than 80% capacity either end up in landfills or are recycled to extract precious metals,” Tang said. “Our goal is to build innovative AI-enabled battery performance metrics that will allow car owners to easily assess the health of their EV’s battery but also help establish a new ecosystem to quickly identify and repurpose gently used cells for less energy-intensive applications.”